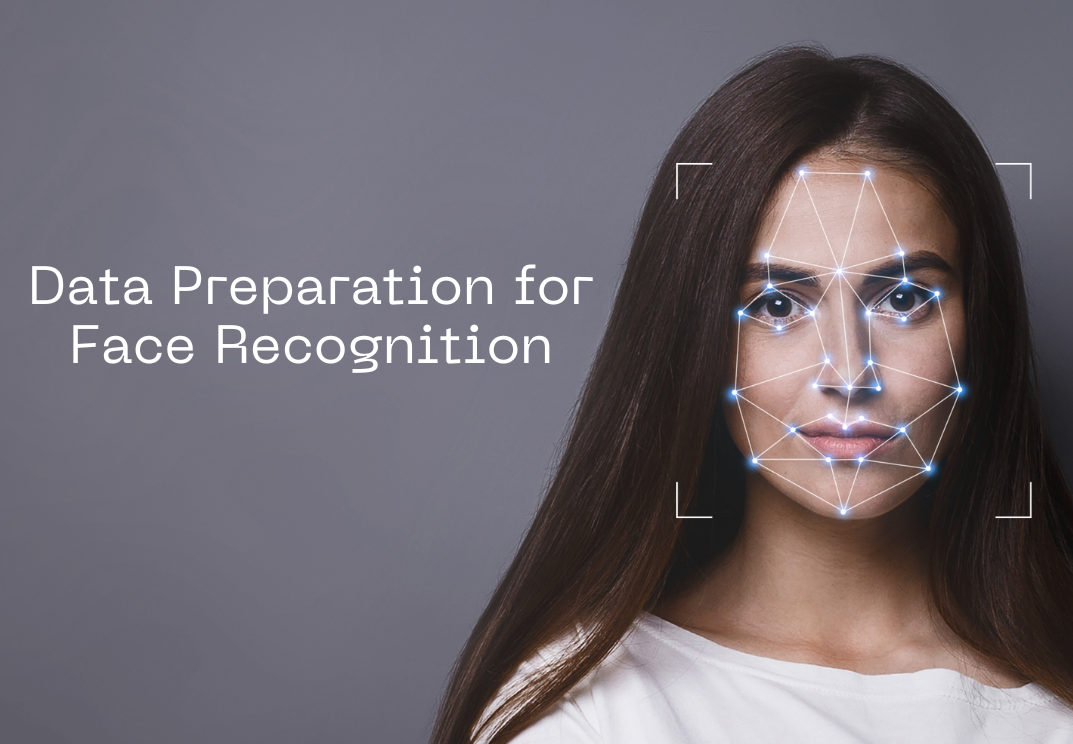
Data Preparation: The Foundation of Accurate Face Recognition
In today's rapidly advancing tech world, we've witnessed incredible progress that's turned futuristic ideas into tangible reality. One key player behind this innovation is Deep Learning, an aspect of AI that mimics how our brains process information to make decisions. It's like a smart subset of machine learning that learns from data without being specifically taught. Deep Learning powers a bunch of amazing things we use every day, like self-driving cars and virtual assistants. In this blog, we're diving into how it makes facial recognition possible.
What is Face Recognition?
Face recognition is a technology that identifies or verifies a person's identity by analyzing their facial features. It's like how we recognize someone by their face. Computers use special algorithms to do this by analyzing images or video frames to determine if the face matches known faces in a database. Within the realm of computer vision, it stands as one of the most crucial applications, garnering substantial attention in commercial domains. Both static and real-time face recognition methods constitute areas of extensive study within computer vision, offering broad applications across numerous fields, including security, surveillance, law enforcement, biometrics, service industries, marketing, and beyond.
This technology is closely tied to face detection, a preliminary process responsible for identifying, localizing, and extracting the facial region from an image background. Once the face is isolated, specific face recognition methods are applied to establish an individual's identity based on their facial characteristics.
How Does Face Recognition Work?
Face recognition methods utilize diverse techniques for authenticating and identifying individuals through facial features. This biometric technology operates by scrutinizing unique facial patterns, providing a non-intrusive approach for identity verification in security, finance, and everyday applications. Unlike typical object classification tasks, face recognition encounters distinctive challenges owing to the intricacies of facial features. It involves handling numerous classes (individual faces) with subtle differences between them and significant variations within the same individual due to factors like pose, lighting, expressions, age, and occlusions.
Deep learning, a subset of machine learning, plays a vital role in modern face recognition approaches. These methods employ deep neural networks that learn multi-level representations of facial features, capturing various levels of detail. This hierarchical structure enables the models to identify faces effectively, displaying resilience to changes in facial pose, lighting, and expressions.
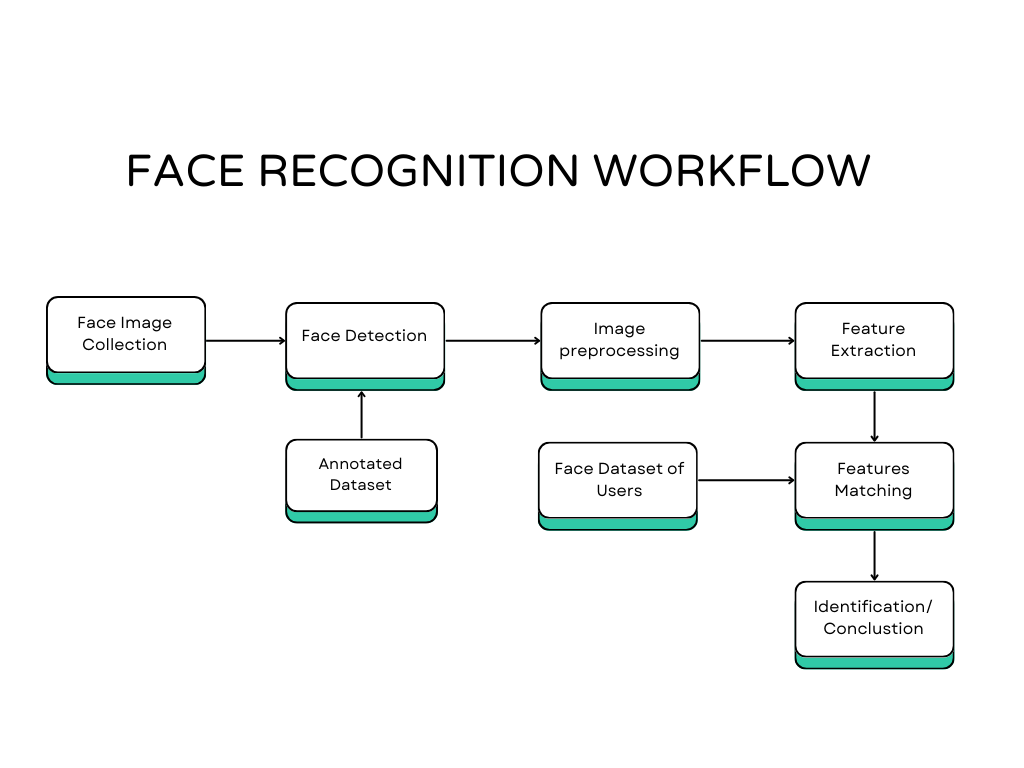
Facial recognition, a technology identifying individuals via their facial features, operates through several key processes:
Face Detection: Begins by identifying faces within images or videos, a common feature in many cameras and social media apps.
Face Alignment: Normalizes facial orientation, ensuring consistency for easier comparisons by algorithms.
Measurement and Extraction: Gathers and extracts various facial features to facilitate comparison with stored data.
Face Recognition: Utilizes deep learning algorithms to match collected facial measurements with known faces in a database.
Face Verification: Confirms the match between the queried face and the database, a crucial step in the process.
Data Preparation for Face Recognition
Data preparation stands as the cornerstone of a robust face recognition solution. It's the essential first step in ensuring accurate and effective facial recognition technology. Within this crucial process, each step holds its significance, from collecting and curating the right image datasets to meticulously annotating and cleaning the data. The subsequent explanation of each step delves deeper into how meticulous data preparation sets the stage for a successful and reliable facial recognition system.Here's a more detailed explanation of the steps involved in preparing data for facial recognition:
Data Acquisition:
Data Collection Planning: Establish a detailed plan outlining specific requirements and intended uses for the facial recognition system. This involves defining demographic profiles, diversity parameters, and variations in facial attributes necessary for training a robust model.
Data Sources and Acquisition: Identify and collect datasets that match the defined criteria. Sources can include publicly available databases, open-source datasets, or custom data collection methods involving the use of cameras, images from the web, or proprietary databases. Acquire images that represent diverse demographics, different ethnicities, age groups, genders, and facial variations to cover a wide spectrum of scenarios.
Data Curation & Cleaning:
Data Scrubbing and Quality Control: Conduct a comprehensive review and cleaning process to remove irrelevant, low-quality, or duplicate images from the acquired datasets. This step ensures that the dataset comprises high-quality, relevant images for effective model training.
Preprocessing Tasks: Implement preprocessing techniques such as image resizing, normalization, noise reduction, and contrast enhancement to standardize the dataset and improve image quality. This step optimizes the dataset for model training by minimizing noise and inconsistencies.
Metadata Preparation:
Metadata Annotation: Assign metadata tags to each image and face within the dataset. These metadata tags include critical facial attributes like yaw, pitch, roll angles, age, gender, ethnicity, skin tone, facial expressions, and other relevant parameters.
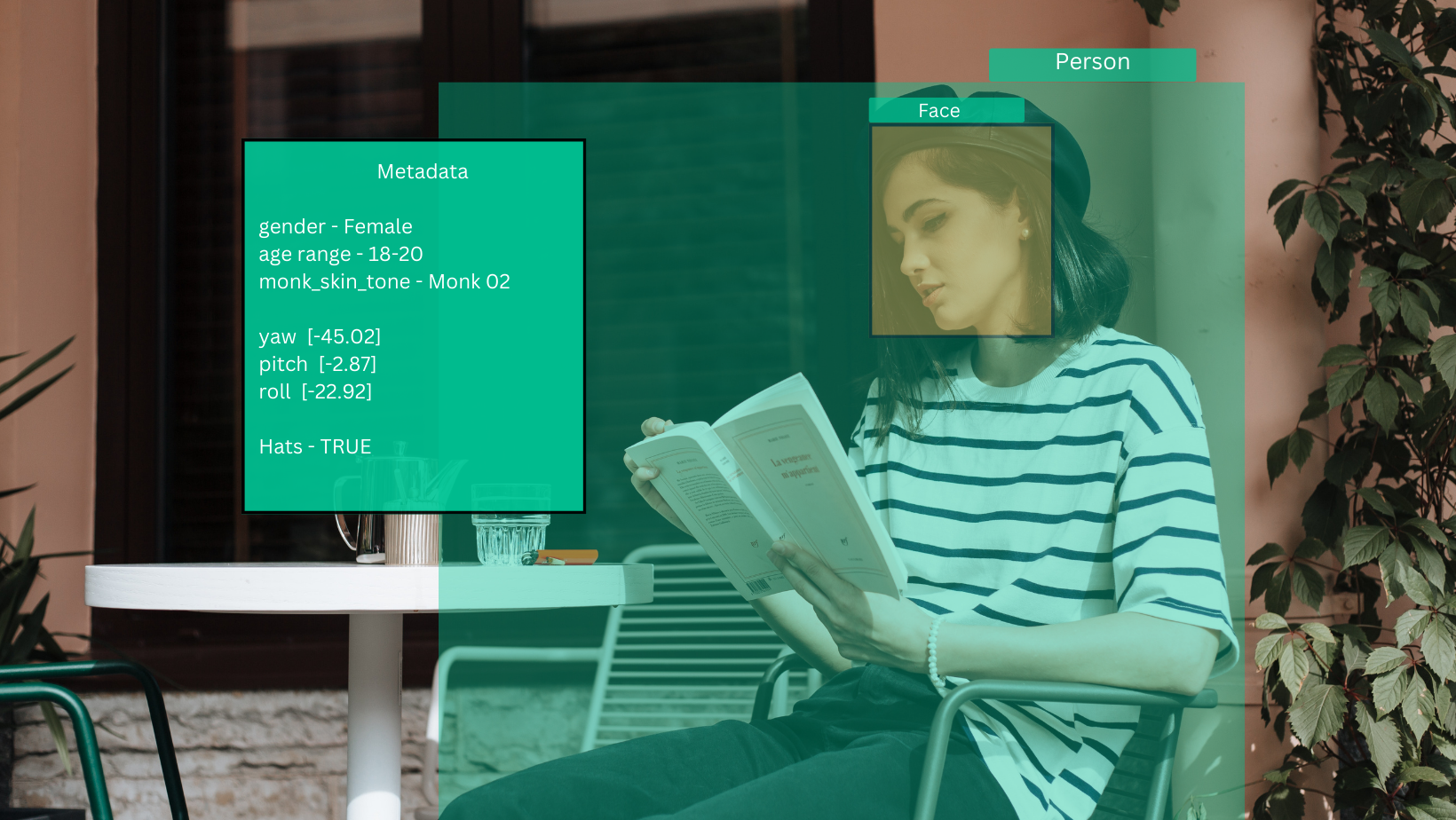
Standardization of Metadata: Ensure uniformity and consistency in metadata annotation across the entire dataset. Consistent metadata annotation facilitates effective training and analysis of facial recognition models by providing accurate information about facial attributes.
Data Annotation:
Facial Annotation: Utilize advanced annotation tools and techniques to label the face and crucial facial landmarks, expressions, gestures, and emotions within the images. This meticulous annotation process involves accurately marking key facial features essential for training facial recognition algorithms.
Annotation Quality Assurance: Ensure high-quality annotations by employing manual or automated annotation methods. This process ensures precision and consistency in labeling facial attributes throughout the dataset, minimizing errors and discrepancies in the annotated data.
Characteristics of good training dataset
A good training dataset for facial recognition should possess several key characteristics to ensure the development of accurate and robust facial recognition models:
- Diversity and Representativeness: The dataset should encompass a wide spectrum of facial variations, covering diverse demographics, ethnicities, age groups, genders, skin tones, and facial expressions. This diversity ensures that the model learns to recognize and differentiate faces across different characteristics, avoiding bias and improving generalization.
- Quality and Consistency: High-quality images with consistent resolutions, clear facial features, and minimal noise are crucial. Consistency in lighting conditions, image orientations, poses, and facial expressions across the dataset is essential for effective training. This quality ensures that the model learns relevant features without being influenced by noise or variations.
- Balanced and Adequate Volume: A well-balanced dataset with an adequate volume of samples for each class or category (age groups, ethnicities, genders) ensures that the model receives sufficient exposure to all facial variations. Insufficient or imbalanced datasets can lead to biased models that perform poorly on underrepresented groups.
- Annotation and Labeling: Accurate and detailed annotations of facial attributes, including key facial landmarks, expressions, gestures, and emotions, are essential. Properly labeled datasets enable the model to learn distinct facial features and variations, enhancing its ability to recognize and differentiate faces accurately.
- Ethical and Privacy Compliance: Compliance with ethical guidelines and privacy regulations is critical when curating facial recognition datasets. Respect for individuals' privacy rights, obtaining necessary consent for data usage, and ensuring anonymization of personal information are vital considerations.
- Realistic and Varied Scenarios: Datasets reflecting real-world scenarios, encompassing different environments, camera angles, occlusions, and facial occlusions (glasses, facial hair) enhance the model's adaptability to various conditions and scenarios.
- Preprocessing and Standardization: Preprocessing techniques like image normalization, alignment, and standardization ensure uniformity and consistency in the dataset. This preprocessing optimizes the data for effective model training, reducing noise and irrelevant variations.
- Adaptability and Generalization: A dataset that allows the model to adapt and generalize well to unseen or new facial variations is crucial. A model trained on a dataset that can generalize effectively performs better when applied to real-world applications and diverse user groups.
- Continuous Updates and Maintenance: Regular updates and maintenance of the dataset are essential to keep the model up-to-date with evolving facial characteristics, trends, and technology changes.
TagX: Your trusted Data partner
While facial recognition and machine learning possess imperfections, their research and application continue to thrive for academic and other purposes. In today's digital landscape, ensuring the safety and security of facial recognition technology remains a critical concern for governments, legislators, and developers alike.
In the realm of data preparation for such systems, specialized firms play a pivotal role in streamlining workflows, eliminating the laborious tasks of sorting, cleansing, and classifying datasets. Recognizing the intricate nuances of employing machine learning in facial recognition, TagX offers top-tier, secure data labeling solutions that adhere to stringent industry security standards. Furthermore, our hardware, software, and data labeling processes strictly comply with GDPR regulations, ensuring robust security measures.
In the domain of facial recognition, the quality and reliability of data stand as the bedrock of success. At TagX, our commitment to delivering dependable data for facial recognition systems is unwavering. We understand that accurate and well-labeled datasets are the cornerstone of efficient machine learning models. With a meticulous approach, we curate, clean, and annotate data, ensuring that each dataset meets the highest standards of accuracy and relevance. Our expertise in data labeling for facial recognition spans a wide spectrum, encompassing diverse facial poses, lighting conditions, expressions, and demographic variations.