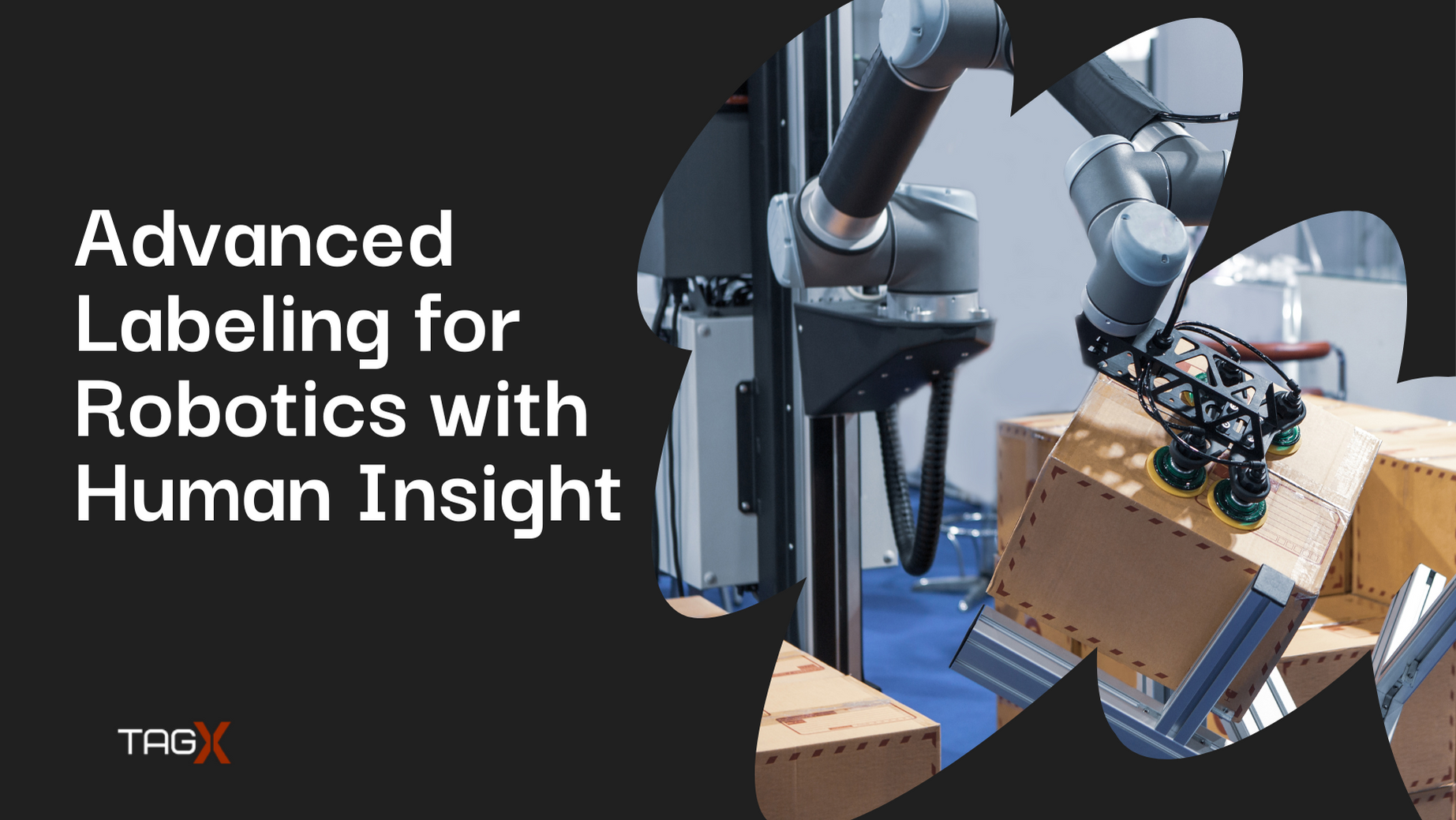
Precise automated labeling with human insight for Robotics
One of the main application of AI is robotics which is becoming accessible across a number of industries all around the world. Robots and robotics has been part of various industries and economical areas for years but its accessibility has been quite limited to a few sectors. Now with the emergence of AI we have the real opportunity to integrate robotics in industry, something we didn’t dare to imagine could be possible just months ago.
Within the quickly changing field of robotics, artificial intelligence has become a key factor in efficiency and creativity. Specifically, automated labeling has completely changed how robotic systems process and use data. In robotics, automated labeling is essential for tasks like data annotation, semantic segmentation, and object recognition, which allow robots to successfully perceive and interact with their surroundings. By using AI robots are able to recognize objects, comprehend scene layouts, and acquire knowledge. In this way robots and robotics in general can undertake difficult and complex work and achieve precision in their everyday tasks.
Furthermore, automated labeling helps to generate synthetic datasets for training in simulated environments, enhances data quality management, and supports processes related to active learning and reinforcement learning. To make it more simple automated labeling is crucial in helping robots in improving their perception and judgment on a variety of processes. But as we embrace automation's advantages, it becomes more important than ever to recognize its limitations and the necessity for safety precautions, particularly in vital fields like robotics.
Automated labeling for Robotics
For well-known reasons, precision is key in robotics. Above all it enhances the credibility across industries and in everyday life. Automated labeling is crucial for data accuracy and dependability in a range of robotic applications. Accurate data labeling is essential for robotic applications that need precise and efficient robot operation, such as autonomous car navigation, surgical robotics manipulation, and item recognition in manufacturing.
Automated labeling systems use advanced algorithms and machine learning techniques to mark vast volumes of data rapidly and precisely. By automating the labeling process, robotics engineers can increase the effectiveness of robotic systems and hasten the development of AI models. Moreover, automated labeling allows robots to learn and adapt continuously, enabling them to become increasingly skilled over time.
Limitations and Risks
Automation does have advantages, but there are also risks involved that need to be properly evaluated. The possibility for errors in automated labeling is one of the main worries. Although algorithms aim for accuracy, they are not perfect and can occasionally misidentify data, producing incorrect results. A small mistake could have serious repercussions in high-stakes applications like driverless cars or medical robotics.
As we may know there are differences between manual and automated labeling starting with scalability. Data labeling by hand takes a lot of effort and is not scalable. The quantity of data that a person may label is limited. Automated data labeling, on the other hand, can save enormous amounts of time.
Adaptability and governability being the two other major differences. When new error modes or data drifts are discovered, or when requirements change, training sets need to be relabelled. Time and resources are wasted because manual data labeling requires many assessments of each data point to stay up to date with changing needs. minimal demands on resources and time.It is challenging to audit the labeling decisions made when data is labeled manually since there is no documentation of the reasoning or conclusions that went into the particular classification of those labels.
Moreover, some kinds of data or situations, such complicated surroundings or unusual events, may be difficult for automatic labeling systems to handle. In certain situations, human involvement could be required to guarantee precise labeling and avoid mistakes. Furthermore, biases in the training data may be unintentionally reinforced by automated labeling systems, producing unfair or biased results.
The human interaction in labeling
A balanced strategy that combines the advantages of automation with human supervision and intervention is needed to overcome these issues. Although data annotation can be accelerated by automated labeling, human specialists are essential for confirming label correctness and spotting potential mistakes. Systems with humans reviewing and correcting automatic labeling, or person-in-the-loop systems, provide a compromise between the accuracy of human judgment and the efficiency of automation.
Moreover, implementing automatic labeling systems in robots requires accountability and transparency. It is imperative for developers to guarantee that consumers comprehend the constraints of automation and are cognizant of the possible hazards that come with depending only on automated procedures. Through cultivating an environment of openness and responsibility, the robotics community can reduce risks and increase confidence.
A recent Forbes article emphasized the fact that a pivotal aspect of ML model development is understanding that we educate them rather than code them. These models function akin to a collective workforce of robots, capable of executing tasks swiftly within hours. It falls upon us, as humans, to provide top-tier training data to continually refine and enhance ML model performance.
TagX your trusted Data partner
Automation must be done carefully and precisely, even though automated labeling has great potential to advance robotics. We can maximize the advantages of automation while lowering risks and guaranteeing the dependability and safety of robotic systems by understanding the limitations of automation and putting preventative measures in place. To advance robots toward a future of safety and creativity, precision and caution are, in fact, two sides of the same coin.
At TagX, we believe in the human-in-the-loop concept, acknowledging that human intelligence plays an essential role in conjunction with machine learning algorithms. Our methodology ensures the highest quality and accuracy in our AI models by integrating human experience at every stage of the data annotation process. We improve the performance of machine learning systems by utilizing human cognitive abilities, which are superior to automated algorithms in certain areas. This concept serves as the foundation for our dedication to provide trustworthy, moral, and socially conscious AI solutions. At TagX, we value the complementary qualities of human and machine intelligence. By enabling businesses to fully utilize artificial intelligence, we uphold openness and accountability.